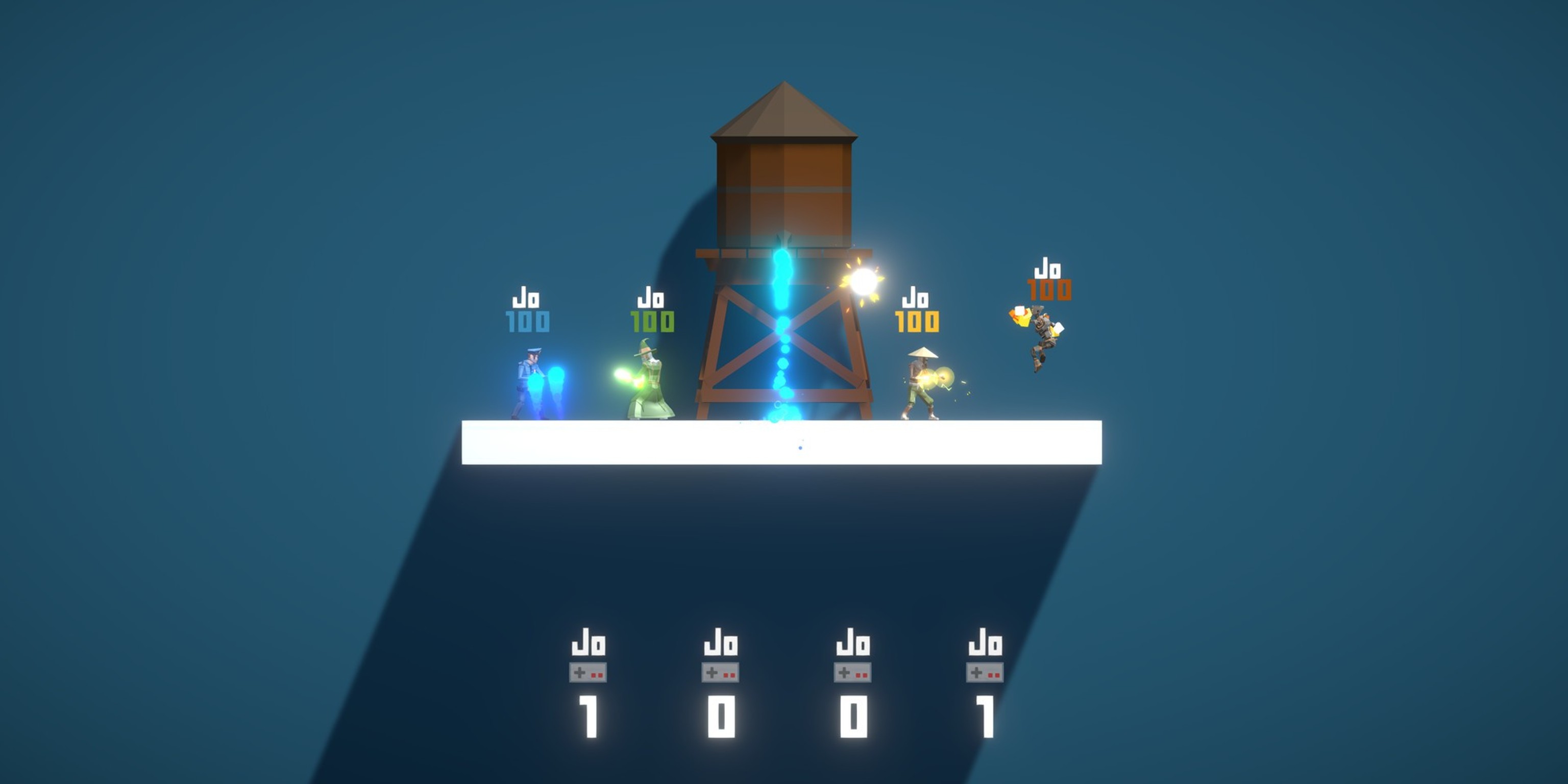
Long-term motivation effects of deep player behavior models for dynamic difficulty adjustment
Machine Learning
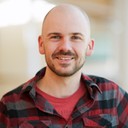
Collaborators Digital Media Lab, University of Bremen
Abstract
This study looks at the effects of deep player behavior modeling (DPBM) via dynamic difficulty adjustments (DDA) as compared to heuristic parameter tuning (HPT) on intrinsic motivation, perceived challenge and player motivation in a real-world MMORPG.
Method
A purpose-built online multiplayer game online Korona:Nemesis, was released on Steam for this study.
Takeaways
Players prefer game experience episodes featuring DPBM over experience episodes with classic difficulty management.
Balancing games and producing content that remains interesting and challenging is a main cost factor in the design and maintenance of games. Dynamic difficulty adjustments (DDA) can successfully tune challenge levels to player abilities, but when implemented with classic heuristic parameter tuning (HPT) often turns out to be very noticeable, e.g. as “rubber-banding”.
Deep learning techniques can be employed for deep player behavior modeling (DPBM), enabling more complex adaptivity, but effects over frequent and longer-lasting game engagements, as well as how it compares to HPT has not been empirically investigated.
We present a situated study of the effects of DDA via DPBM as compared to HPT on intrinsic motivation, perceived challenge and player motivation in a real-world MMORPG. The results indicate that DPBM can lead to significant improvements in intrinsic motivation and players prefer game experience episodes featuring DPBM over experience episodes with classic difficulty management.
A purpose-built online multiplayer game online Korona:Nemesis, was released on Steam for this study.
Takeaways
We presented the design and implementation of an adaptive instance dungeon in the MMORPG Aion to evaluate a novel, implicit take on Dynamic Difficulty Adjustment that is not dependent on manually composed parameter tuning, but affords a continually adapting challenge through Deep Player Behavior Modeling.
In an extensive medium-to-long-term study (n = 171 over the course of four weeks) we contrasted an opponent applying DPBM to traditional DDA parameter tuning and can report significantly higher intrinsic motivation stemming from the unique game experience of being confronted with strategic behaviors that mirror one’s own patterns.
Qualitative statements reinforce the approval and positive experience of DPBM, while the consistent and dominant usage of the instance throughout the whole study period reflects its potential to elevate long-term motivation and commitment.
Regarding the technical applicability of the approach, we report on the DPBM architecture, its accuracy and data structure and give an estimation about the temporal demand, yielding real-time potential.